Marina Jimenez Munoz, a PhD student in statistics from the School of Mathematics, Statistics and Actuarial Science (SMSAS) at the University of Kent, was selected as the winner of the Silver Award for Mathematical Sciences at the STEM for Britain event at the House of Commons last night (Wednesday 13 March).
Marina (pictured above on the right) received the award of £1,250 for her research poster (right) exploring the variations in bird populations across Britain as part of her research within the Statistical Ecology at Kent group.
Abstract:
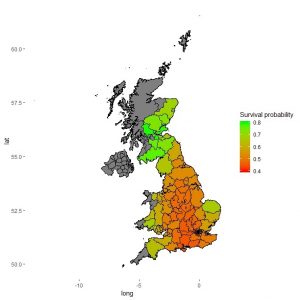
To mitigate biodiversity loss, we need to understand the environmental and demographic causes of changes in the distributions and abundances of species. Bird populations are in a continual state of flux; these fluctuations can be explained by changes in vital rates, such as survival and productivity (breeding success). The British Trust for Ornithology (BTO) collects data through several complementary schemes. These schemes include those recording data on uniquely identifiable animals (ringing data) (Jiménez-Muñoz et al., 2018), and on counts of the number of animals observed (census data). While census data are used to estimate abundance, ringing data are used to estimate survival probabilities. Integrated population models (IPMs) combine these two types of data into one single analysis, making it possible to also estimate productivity, which is unobtainable from either type of data alone. Additionally, results from IPMs are more reliable than those derived from separate analyses (Abadi et al., 2010). IPMs are typically fitted using the Kalman Filter, which is computationally efficient but relies on Gaussian approximations.
Animals are affected by local environmental conditions that vary with space. However, until now, IPMs have either ignored spatial information or divided Britain into two or three areas (e.g. North/South) (Robinson et al., 2014). Thus far, my research has involved incorporating detailed local spatial information, such as geographical coordinates and habitat type data, into IPMs. In so doing, we have also developed, for the first time, spatially-explicit models for ringing and census data alone. Spatial census data are particularly challenging to model because of the presence of very small counts, which violates the Gaussian approximation of the Kalman Filter. To address this issue we have instead considered Hidden Markov models, which can make use of very small counts, as their validity does not rely on Gaussian approximations. Furthermore, since different data schemes collect and register data at different geographical scales we have also developed techniques for reconciling spatial data at different resolutions.
We illustrate our models using data on Starling, Sturnus vulgaris, a species on the RSPB’s red list (of highest conservation priority) in the UK, collected by the BTO. Figure 1 shows estimates of spatially explicit survival probabilities for Starlings (areas where no data were collected are in grey), for the years 1990 to 1999. These results are based on an IPM that uses a quadratic curve to quantify the impact of latitude and longitude on survival. We use these results to demonstrate the need for spatial information in ecological models, and more specifically IPMs. We further complement these with other types of spatial data, such as habitat.
Our models allow for finer analyses within a country and provide robust spatial results that can explain demographic changes in populations caused by geography, weather conditions and/or land type. These models could impact conservation policies by helping experts better identify the particular areas of Great Britain where specific species have low survival. These could be prioritized for interventions, such as habitat protection or restoration. Ultimately this would result in a more efficient allocation of Governmental financial resources and in the improvement of species conservation and biodiversity management.
References
Abadi, F., Gimenez, O., Arlettaz, R., and Schaub, M. 2010. An assessment of integrated population models: bias, accuracy, and violation of the assumption of independence. Ecology, 91:7-14.
Jiménez-Muñoz, M., Cole D. J., Freeman S. N., Robinson R. A., Baillie S. R., and Matechou E. (Accepted, awaiting publication). Estimating age-dependent survival from age-aggregated ringing data – extending the use of historical records. Ecology and Evolution.
Robinson R. A., Morrison C., and Baillie S. R. 2014. Integrating demographic data: towards a framework for monitoring wildlife populations at large spatial scales. Methods in Ecology and Evolution, 5: 1361-1372.